报告人:Xiaojun Zhai
时间:8月8日上午10:00
地点:文津楼3524
主办单位:永利yl23411 普适计算团队
报告标题:Efficient and adaptive computing for AI and Robotics
摘要:
Deep learning (DL) is the key technique in modern artificial intelligence (AI) that has provided state-of-the-art accuracy on many applications. Today, although most of the computational loads of DL systems are still spent running neural networks in data centres, the ubiquity of smartphones, and the upcoming availability of self-contained wearable devices for augmented reality (AR), virtual reality (VR) and autonomous robot systems are placing heavy demands on DL-inference hardware with high energy and computing efficiencies along with rapid development of DL techniques. This demand is in line with the recent surge of edge computing, where raw data are processed locally in edge devices using their embedded inference algorithms; it is expected that there will be a significant growth in edge AI inference in the future. Recently, we have witnessed a distinct evolution in the types of DL architecture, with more sophisticated network architectures proposed to improve edge AI inference. This includes dynamic network architectures that change with each new input in a data-dependent way, where inputs and internal states are not fixed. Although such new DL architectural concepts have demonstrated a great potential to innovate current DL techniques, they are likely to affect the type of hardware and software that will be required to deliver such capabilities efficiently in the future. We address this challenge and propose to design a new flexible hardware architecture to enable adaptive support for a variety of DL algorithms on embedded devices. To produce lower cost, lower power and higher processing efficiency DL-inference hardware that can be dynamically configured for dedicated application specifications and operating environments, this will require radical innovation in the optimisation of network architecture, software and hardware of current DL techniques.
报告人介绍:
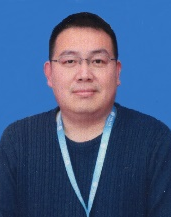
Dr Xiaojun Zhai is a Senior Lecturer with the School of Computer Science and Electronic Engineering (CSEE) at the University of Essex, where his primary research focus is on embedded systems and System-on-Chip (SoC) architecture, with focus on intelligence, performance, security, power, and reliability. He has successfully secured a sustained and increasing trajectory of significant external research funding in my career (in average of £229,344 per annum between April, 2020-June, 2024), including 4 major EPSRC funded projects (in total about £3M) as well as 6 Innovate UK research projects (in total £600K), and one highly competitive grant from Royal Society (£12K). So far in his research career he has been involved as the PI/Co-leader/Key researcher in a number of international and national research projects, including EPSRC EP/V034111/1, EP/X019160/1, EP/V000462/1, RoBoSAS: EP/K004638/1, National Centre for Nuclear Robotics (NCNR): EP/R02572X/1, FP7 SWIPE, Interreg SYSIASS, and INCASE, as well as the Qatar National Priorities Research Program (NPRP) with EMBIoT, and CER2EBRAL. He has successfully co-supervised 3 PhD students, and is involved in day-to-day management and supervision in the Embedded and Intelligent Systems (EIS) laboratory, where he leads one EPSRC project, 5 PhD student projects and co-supervises 4 post-doctoral researchers. His work to date has resulted in more than 120 scientific journal and conference publications, including IEEE Transactions on IFS, IEEE Transactions on SMC: Systems, IEEE Sensors/System Journal, ACM Transactions on IST, as well as several leading international conferences, such as CVPR, FCCM, ICIP.